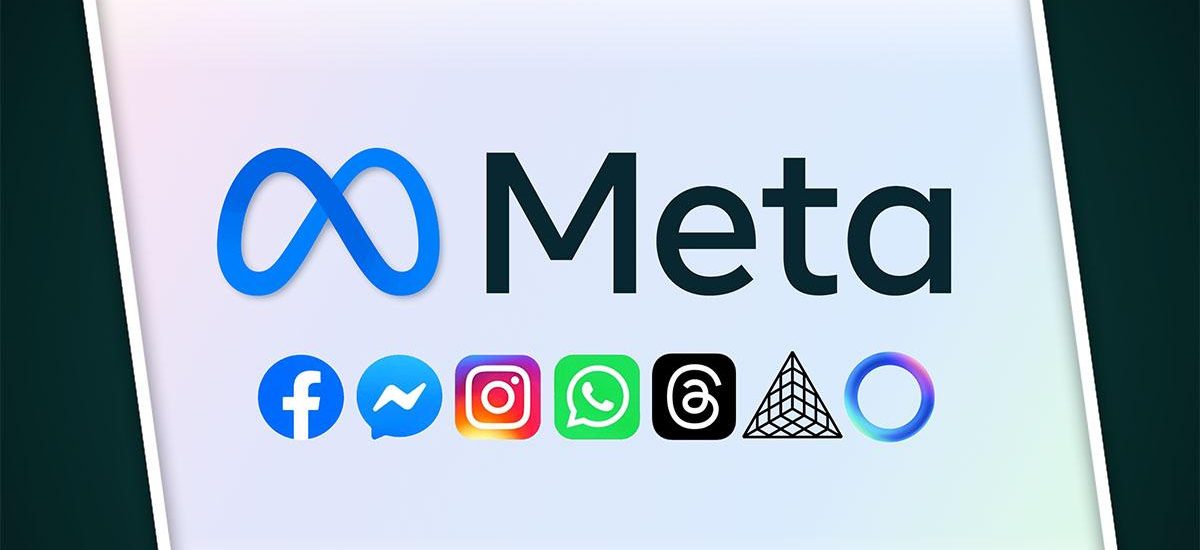
In the ever-evolving landscape of artificial intelligence, where headlines often tout breakthroughs and innovations, a provocative assertion from one of the industry’s leading minds has sparked contemplation. Meta’s chief AI scientist has recently asserted that simply scaling AI—the popular strategy of expanding model size and data—won’t necessarily lead to a notable increase in intelligence. This statement challenges widely-held beliefs about the path to smarter machines and invites a deeper examination of what true intelligence in AI entails. As we delve into the nuances of this viewpoint, we uncover the complexities of AI growth and the critical dialog it inspires within tech circles and beyond.
Understanding the Limitations of Scaling AI in Enhancing Intelligence
The allure of scaling artificial intelligence often leads to the assumption that an increase in computational resources and data will directly enhance cognitive abilities. However, this perspective overlooks crucial factors that define intelligence itself. Core limitations exist that impede the progress toward more intelligent AI,regardless of scale. These include:
- Lack of contextual understanding: Current AI systems struggle to grasp nuances, sarcasm, and emotional contexts.
- Data biases: Scaling up means amplifying existing biases in training datasets, which can lead to flawed decision-making.
- Complexity of intelligence: Human intelligence encompasses creativity, intuition, and emotional insight, areas where AI still falls short.
Moreover, as systems expand, they frequently enough become more intricate, introducing new challenges that can stymie innovation rather than foster it. Such as, increased parameters may lead to diminishing returns in performance, where the effort invested yields minimal gains. A comparative analysis often illustrates this trend:
Model size | Performance Gain | Diminishing Returns Indicator |
---|---|---|
Small | High | Significant advancement |
Medium | Moderate | Reduced progress |
Large | Low | Minimal enhancement |
This chart illustrates that simply increasing the model size dose not guarantee proportional improvements in capabilities, highlighting the paradox inherent in rapid scalability. Thus, while the technological infrastructure expands, the fundamental nature of intelligence remains complex and multifaceted, frequently enough unyielding to straightforward scaling methods.
the Role of Data Quality Over Quantity in AI development
In the realm of artificial intelligence, the old adage “more is better” doesn’t always hold true. The significance of data quality has emerged as a critical factor that can determine the success of AI systems. Powerful algorithms trained on vast datasets can yield extraordinary outputs, but if the underlying data is flawed, biased, or irrelevant, the results can be misleading or even detrimental. High-quality data ensures that AI can not only learn effectively but also make accurate predictions, thereby enhancing its utility across diverse applications. To illustrate this notion, consider the following key aspects of data quality:
- Accuracy: Data should reflect the real world correctly.
- Completeness: Datasets must include all necessary information for a comprehensive view.
- Consistency: Information should be reliable across various sources.
- Relevance: Data must pertain to the specific problem being addressed.
Moreover, the pitfalls of prioritizing quantity over quality can lead to significant issues, highlighting the importance of a strategic approach to data curation. Large datasets often come with noise—irrelevant or duplicate entries—that can cloud the insights AI seeks to derive. A well-structured dataset can be far more valuable than a massive one fraught with inconsistencies. The following table encapsulates the contrasts between high-quality and low-quality datasets:
Characteristics | High-Quality Data | Low-Quality Data |
---|---|---|
Impact on AI Performance | Improved accuracy and reliability | Misinformation and errors |
Training Efficiency | Faster convergence | Slower training due to noise |
User Trust | High user confidence | Low user trust |
Integrating Human Expertise: A Path to Smarter AI Solutions
In the quest for more intelligent AI systems, the age-old debate surrounding the synergy between human expertise and artificial intelligence continues to gain momentum. As Meta’s chief AI scientist suggests, simply scaling algorithms and data may not yield the smarter solutions we seek. Rather, a multi-faceted approach that integrates human knowledge into the AI training and evaluation process is essential. By harnessing the critical thinking and contextual understanding that only humans possess, we can fine-tune AI systems to navigate complex scenarios more effectively.
Consider a collaborative model where AI functions as an extension of human capabilities,rather than a replacement. This model invites contributions from various fields, leading to more robust insights. Some potential benefits include:
- enhanced Decision-Making: combining human intuition and judgment with AI’s computational prowess.
- Improved Accuracy: Reducing biases by engaging diverse expert opinions during AI training.
- Real-World Application: Tailoring AI solutions by grounding them in human experiences and social contexts.
To visualize this collaboration, the following table outlines key areas where human expertise can enhance AI development:
Area of Integration | Human Contribution | AI Enhancement |
---|---|---|
Data Annotation | Contextual understanding of nuanced categories | Higher quality labeled datasets |
model evaluation | Critical analysis and feedback | More reliable performance metrics |
Ethical Considerations | Awareness of societal impacts | Responsible AI usage |
Future Directions for AI Research: Fostering Innovation Beyond Scale
As the landscape of artificial intelligence evolves, it’s crucial to explore avenues that transcend mere scaling. While increasing data and computational power have historically been the primary drivers of AI development, there needs to be a shift towards cultivating deeper insights and understanding in algorithms. innovative methods like cross-disciplinary approaches and ethical AI practices are becoming indispensable for creating intelligent systems that not only perform tasks but also comprehend the implications of their actions. Key strategies for fostering this innovative spirit include:
- Exploring interdisciplinary Collaboration: Bringing together expertise from fields such as neuroscience, psychology, and philosophy can enrich AI’s development.
- Implementing Robust Ethical Frameworks: Developing guidelines that govern AI interactions can ensure more responsible outputs.
- Focusing on Interpretability and clarity: Creating AI that users can understand and trust is essential for greater adoption and efficacy.
In addition to exploring these innovative paths, a nuanced understanding of AI’s potential limitations must guide future research. Customary metrics that prioritize raw processing power can overshadow the need for systems that mirror human-like reasoning and problem-solving capabilities. Next-generation AI can benefit from techniques such as:
Technique | Description |
---|---|
Causal Inference | Understanding cause-and-effect relationships to improve decision-making. |
Meta-Learning | Teaching AI to adapt and learn from new tasks with minimal data. |
Human-AI Collaboration | Creating systems that work synergistically with humans, enhancing creativity and productivity. |
Wrapping Up
the insights from Meta’s chief AI scientist shed light on a critical distinction in the world of artificial intelligence: scaling alone is not the silver bullet to achieving true intelligence. As the technology evolves, it’s essential to recognize that depth, nuance, and understanding are not merely products of size. The conversation surrounding AI’s future must shift toward exploring how we enrich these systems cognitively, rather than just numerically. By fostering innovative approaches and prioritizing qualitative improvements, we can guide AI development towards becoming not just larger, but also smarter in ways that are meaningful and beneficial to society. As we stand on the precipice of technological advancement, the journey towards smarter AI will require a thoughtful blend of creativity, ethics, and responsibility—one that extends beyond the confines of scaling. The future of AI is not just about numbers; it’s about wisdom, understanding, and the ways in which we choose to engage with this powerful tool.