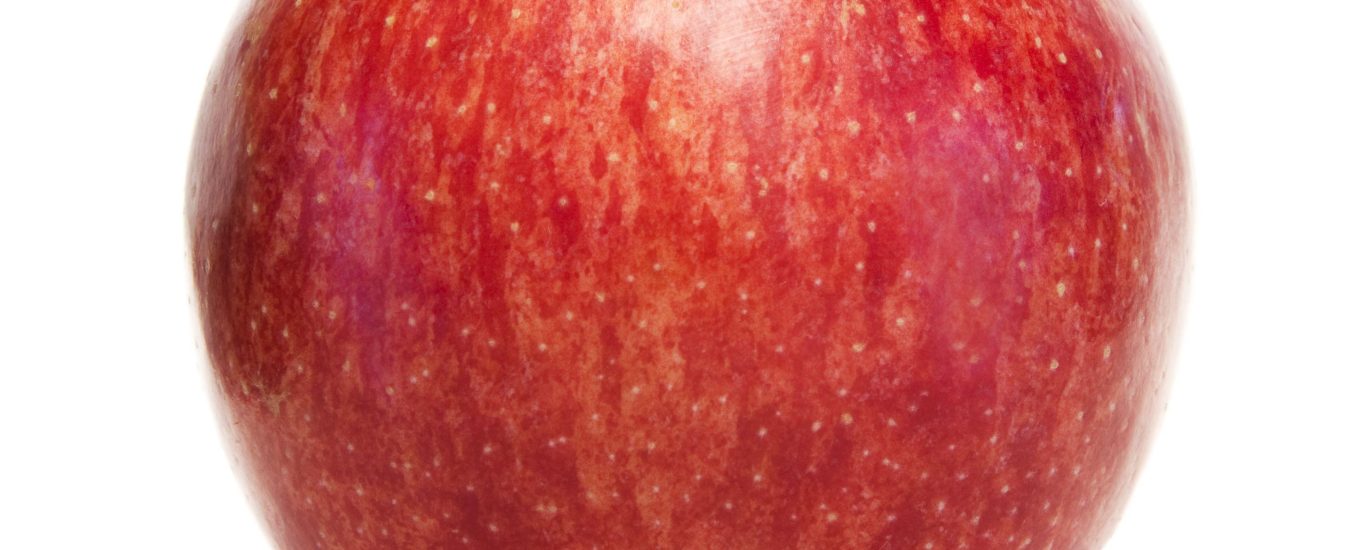
In an era where artificial intelligence is reshaping the way we interact with technology, the nuances of algorithmic performance are more crucial then ever. Recently, a leaked document has emerged from the corridors of Apple, unveiling the company’s preference ranking guidelines for AI-generated responses. This insider look into Apple’s scoring system not onyl sheds light on the criteria that govern the delivery and refinement of AI content but also raises compelling questions about openness, accountability, and the ever-evolving relationship between humans and machines.As we delve into the intricacies of this scoring system, we will explore its implications for AI advancement, user experience, and the future of smart interactions in our daily lives.
Understanding Apple’s Scoring Framework for AI-Generated Content
Apple’s scoring framework for AI-generated content is a complex yet systematic approach designed to evaluate the relevance and quality of AI responses. At the core of this framework are specific criteria that help ensure consistency and reliability in the outcomes produced by AI systems. The framework emphasizes the importance of contextual understanding, whereby the AI must not only provide accurate information but also tailor its responses based on the user’s intent. Furthermore, the framework seeks to prioritize user engagement and satisfaction, recognizing that AI content must resonate effectively with the audience it aims to serve.
Central to the scoring process are several key dimensions that influence how responses are rated. These dimensions include but are not limited to: accuracy, relevance, clarity, and originality. Each dimension is meticulously assessed through a scoring rubric, allowing for a nuanced understanding of AI outputs. The table below outlines these dimensions alongside their importance ratings in the scoring model:
Dimension | Importance Rating |
---|---|
Accuracy | 5/5 |
Relevance | 4/5 |
Clarity | 4/5 |
Originality | 3/5 |
Key Criteria for Evaluating AI responses: Insights from the Leaked Document
In the quest to refine AI-generated responses, a set of key criteria has emerged that underscores the effectiveness and quality of these outputs. These benchmarks focus on the relevance of the response to the user’s query, ensuring that the AI maintains contextual awareness. Additionally, factors such as clarity and conciseness are crucial; the ideal response should be easily comprehensible and to the point, avoiding any unnecessary verbosity that might confuse users. Moreover, engagement and creativity play a significant role, as responses that incorporate unique perspectives or insights tend to hold users’ attention more effectively.
To further elucidate these parameters, the leaked document also highlights the importance of accuracy and factual integrity in evaluating AI responses. It emphasizes that responses must not only be relevant but also grounded in reliable information to avoid disseminating misinformation. This leads to a structured scoring system where responses are rated across various dimensions, such as tone appropriateness and user satisfaction. Below is a simplified representation of how different metrics contribute to the overall evaluation:
Criteria | Weight (%) | Description |
---|---|---|
Relevance | 30 | Connection to user’s query |
Clarity | 25 | understanding without confusion |
Creativity | 20 | Unique insights and engagement |
Accuracy | 15 | Factual correctness |
tone | 10 | Appropriateness for audience |
Implications of Apple’s Guidelines on Future AI Developments
The recently leaked guidelines from Apple reflect a significant pivot in how AI-generated content will be evaluated and utilized across various platforms. As major players like Apple define their scoring systems,it paves the way for an increasingly competitive environment for AI developers. The implications are multifaceted, including heightened expectations for the quality of AI outputs, wich must now align with specific scoring metrics that prioritize user-centric relevance. This may encourage developers to refine their algorithms,fostering innovation and a race for higher scoring responses that meet or exceed these new benchmarks.
Moreover, these guidelines may lead to broader industry changes beyond apple, as other companies look to implement similar scoring criteria to remain competitive. As such, AI developers will need to adapt quickly, which could mean investing in more elegant machine learning models or leveraging user feedback more effectively. The following table illustrates potential impacts that Apple’s guidelines could have on future AI directional shifts:
Impact Area | Expected Change |
---|---|
Quality Assurance | Increased focus on accuracy and relevance in AI responses. |
Developer Standards | New benchmarks for AI performance set across the industry. |
User Engagement | Greater emphasis on feedback loops to improve AI interactions. |
Market Dynamics | Potential consolidation of AI firms around those that meet high scoring criteria. |
Best Practices for AI developers in Navigating Preference Rankings
Developers working with AI systems should emphasize a layered approach to aligning their models with preference rankings. It’s crucial to incorporate user feedback at multiple stages of development, ensuring that the AI not only understands preferences but adapts to evolving user expectations. Techniques such as A/B testing can provide insights into how different outputs perform in real-world scenarios, allowing developers to fine-tune response rankings effectively.Incorporating machine learning algorithms that prioritize user engagement metrics can greatly enhance the relevance of AI outputs.
Furthermore, establishing a extensive scoring system based on Apple’s leaked guidelines can help developers systematically assess and refine their AI-generated content. By categorizing responses into various quality markers, such as relevance, clarity, and engagement, developers can create an iterative cycle for improvement. Below is an illustrative scoring table that highlights different response attributes that can be leveraged for evaluation:
Attribute | Scoring Range (1-5) | Description |
---|---|---|
Relevance | 1 - 5 | How closely the response aligns with user intent. |
Clarity | 1 - 5 | Ease of understanding and readability of the response. |
Engagement | 1 – 5 | Level of user interaction spurred by the response. |
This multidimensional scoring framework not only aids in evaluating response quality but also allows developers to set actionable goals for further development, helping to ensure that AI systems remain user-centric and effective.
The Conclusion
In a world where artificial intelligence continues to reshape our interactions with technology, understanding the frameworks that guide its development is more crucial than ever.Apple’s leaked preference ranking guidelines provide a rare glimpse into the scoring system behind AI-generated responses, illuminating the intricate balancing act between user experience and machine learning. As we navigate this evolving landscape, these insights offer not only a valuable understanding of Apple’s approach but also raise significant questions about ethics, accountability, and the future of AI in our daily lives. As consumers and developers alike dive deeper into this realm, staying informed about such guidelines will be essential in fostering a more clear and responsible AI ecosystem. The conversation doesn’t end here; it’s just the beginning.