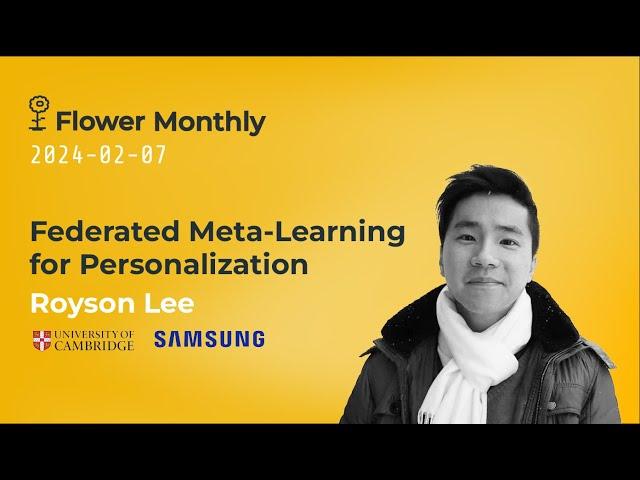
In an era where connectivity reigns supreme, the Internet of Things (IoT) has emerged as a revolutionary force, weaving itself into the fabric of our daily lives. Imagine a world where your devices not only communicate seamlessly but also understand your preferences and behaviors, tailoring their functionalities to serve you better. Enter federated meta-learning, an innovative approach poised to redefine personalized IoT experiences. This article delves into a pioneering framework that harnesses the potential of federated meta-learning, enabling devices to learn collaboratively while respecting user privacy. By shifting the paradigm towards a more adaptive and secure IoT landscape,we explore how this cutting-edge methodology is set to enhance how we interact with our connected world,paving the way for smarter,more intuitive technologies that prioritize both personalization and data protection. join us as we unravel the intricacies of this transformative intersection of federated learning and the Internet of Things, shining a light on the future of personalized technology.
Exploring the Paradigm shift: Personalized IoT and the role of Federated meta-Learning
The convergence of personalized Internet of Things (IoT) with federated meta-learning is revolutionizing how devices interact and cater to individual user needs. By leveraging the collective intelligence of distributed data while maintaining user privacy,this approach enables devices to learn from experiences without compromising sensitive data. Key benefits of this framework include:
- Enhanced user Experience: Devices adapt based on personal preferences, leading to smarter homes and optimized resource management.
- Privacy Preservation: User data stays on the device, allowing for machine learning without the risk of data breaches.
- Dynamic learning: Continuous updates occur as users interact with their devices, ensuring relevance over time.
Federated meta-learning, in this context, acts as the catalyst for seamless collaboration among devices. It orchestrates the training process, aggregating insights from multiple sources while ensuring that individual data sets remain decentralized. the implications of this methodology are vast, including:
Advantages | Challenges |
---|---|
Scalability across diverse devices | Complexity in model synchronization |
Reduction in latency through localized learning | Dependence on device availability |
Improved adaptability to user behavior | Potential for data variance |
Harnessing data Wisely: Security and Privacy in Decentralized Learning environments
In an era where vast amounts of data are generated daily, leveraging this information without compromising security and privacy is paramount. Decentralized learning environments offer an innovative approach by allowing devices to learn collaboratively while maintaining localized data storage. By employing techniques like federated learning, individual devices can improve their algorithms by participating in a distributed network, ensuring that sensitive personal information is never transmitted or exposed. Key strategies to enhance security in this context include:
- Data Anonymization: Techniques to mask identities before processing.
- Strong Encryption: Protecting data-at-rest and in-transit with robust encryption protocols.
- Access Control: Implementing strict authentication measures to limit data access.
additionally, the ethical considerations surrounding data usage in these systems cannot be overlooked. Building a framework for responsible data governance within decentralized learning mandates transparent policies and user consent. Stakeholders must collaborate to ensure users are adequately informed about how their data is utilized, leading to improved trust and engagement. A simplified table illustrating potential data governance principles can aid in understanding:
Governance Principle | Description |
---|---|
Openness | Clear dialog regarding data collection and usage. |
Accountability | Establishing ownership and obligation for data protection. |
Fairness | Ensuring equitable treatment of all data subjects in decision-making. |
Optimizing Device interactions: Strategies for Seamless Integration in Personalized IoT Frameworks
In the rapidly evolving landscape of interconnected devices, optimizing interactions between multiple IoT systems is crucial for achieving a truly personalized experience. By leveraging federated meta-learning, devices can learn collaboratively while maintaining data privacy and minimizing latency. This innovative approach allows for the aggregation of local insights, paving the way for improved adaptability and responsiveness. Key strategies to enable seamless integration include:
- Context-Aware Learning: Devices should utilize environmental data to tailor their interactions based on user preferences and situational demands.
- Cross-Device Communication Protocols: Standardized protocols can facilitate smoother exchanges between diverse devices, enhancing interoperability.
- Adaptive Resource Allocation: Dynamically adjusting resource usage based on real-time demands ensures devices are always performing optimally.
Moreover, implementing a robust framework that integrates these strategies enables devices to form a cognitive network.By analyzing patterns in user behavior and preferences, IoT systems can generate bright predictions that lead to enhanced user experiences. The following table illustrates the advantages of employing federated meta-learning within personalized iot frameworks:
Advantage | Description |
---|---|
Data Privacy Preservation | Minimizes data exposure by keeping sensitive information on local devices. |
Reduced Latency | Real-time processing allows for quicker responses to user commands. |
personalized Experiences | Enhances user satisfaction through tailored interactions based on learned preferences. |
Future Directions: Recommendations for Stakeholders in the Evolving Landscape of Federated Learning
As the landscape of federated learning continues to evolve,it becomes imperative for stakeholders to embrace a collaborative approach that enhances data privacy while delivering personalized services. Developers and researchers should prioritize the integration of robust security measures in federated meta-learning frameworks to ensure that user data remains encrypted and secure throughout the learning process.Additionally, they must foster open-source collaborations and share knowledge across communities to drive innovation that benefits all parties involved.This will facilitate a more efficient and adaptive learning environment, enabling smarter, more responsive Internet of Things (IoT) devices.
Furthermore, policy makers and business leaders should focus on establishing clear guidelines that promote ethical practices in data usage and algorithm transparency. By creating a balanced regulatory framework, stakeholders can nurture trust among consumers while encouraging the growth of federated learning technologies. This can be supported by investment in educational initiatives to ensure that users understand the value and implications of participating in federated learning. Collaborative platforms should be created to allow stakeholders to share insights and progress, fostering a community-driven approach to resolving challenges associated with personalized IoT systems.
In Conclusion
as we venture further into the era of the Internet of Things, the integration of federated meta-learning presents a transformative opportunity for personalized experiences. This innovative framework not only champions privacy and security but also empowers devices to learn collaboratively, revolutionizing how we interact with technology. As we reflect on the implications of this advancement, it is clear that the future of IoT is not just about connectivity, but about smart, tailored interactions that truly resonate with individual user needs. By harnessing the potential of federated meta-learning, we stand on the brink of a personalized digital landscape that promises to redefine our everyday lives. The journey has just begun,and the horizon is filled with possibilities waiting to be explored.