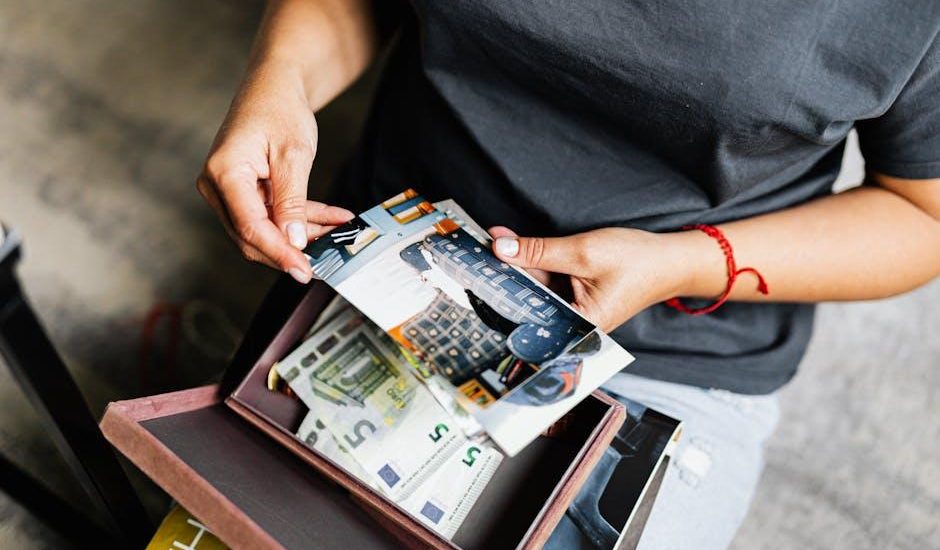
In an ever-evolving landscape of artificial intelligence, where the competition is as fierce as it is innovative, Meta’s newly introduced Maverick AI model has garnered attention—though perhaps not for the reasons the tech giant had hoped. As the dialog surrounding AI continues to expand, so to do the benchmarks by which these models are measured. In a recently released analysis, Maverick’s performance on a widely recognized chat benchmark has revealed a notable gap between it and its competitors. This article delves into the implications of Maverick’s ranking within the context of contemporary AI development, exploring what this means for Meta’s ambitions in the saturated market of conversational agents and the potential challenges that lie ahead. Join us as we unpack the intricacies of this latest AI offering and consider whether it can rise to meet the expectations set by its rivals.
Understanding the Limitations of Meta’s Maverick AI in Chat Benchmark Performance
While Meta’s Maverick AI has garnered attention for its development, a deeper dive into its performance on chat benchmarks reveals significant limitations compared to its competitors. In particular, metrics reflecting user engagement, conversational relevance, and response accuracy highlight areas where Maverick falls short. to illustrate, consider the following factors that contribute to its underwhelming performance:
- Contextual Understanding: Maverick struggles to maintain context across multi-turn conversations, often leading to disjointed exchanges.
- Response Naturalness: While designed to simulate human-like interactions, responses can sometiems feel mechanical or overly formal.
- Topic Adaptability: The model’s ability to shift seamlessly between topics is limited, diminishing the overall user experience.
In comparison to leading rivals,Maverick’s results in standardized chat benchmarks reveal a clear disparity. The following table showcases a snapshot of performance metrics, illustrating how it stacks up against othre AI models:
Model | User Engagement Score | Response Accuracy (%) |
---|---|---|
Maverick AI | 65 | 70 |
competitor A | 85 | 90 |
Competitor B | 80 | 88 |
These figures indicate a critical need for improvement in Maverick’s architecture and training approach to enhance its chat functionality. The challenges highlighted underscore the importance of ongoing research and development in the field of conversational AI, as both user expectations and competitive standards continue to evolve.
Comparative Analysis: How Maverick AI Measures Against Competitors
In the ever-evolving landscape of artificial intelligence,establishing a clear benchmark is crucial for understanding competitive positioning. The recent evaluations have showcased how Maverick AI, developed by Meta, has faced considerable challenges against its counterparts in critical areas. This is especially evident when comparing its performance in natural language processing and user engagement metrics. Notable competitors such as OpenAI’s language model and Google’s Bard have consistently outperformed Maverick AI in the following aspects:
- Response accuracy: Competitors display higher precision in understanding user intent, leading to more relevant answers.
- Context retention: Rivals manage to maintain and utilize conversation history more effectively.
- User satisfaction ratings: Surveys indicate a higher level of engagement and satisfaction from users with alternative models.
To better illustrate this competitive landscape, consider the following comparative performance metrics across several key AI platforms:
AI Model | Response Accuracy (%) | Context Retention (minutes) | User Satisfaction (1-10) |
---|---|---|---|
Maverick AI | 72 | 3 | 6.5 |
OpenAI | 88 | 6 | 9.1 |
Google Bard | 85 | 5 | 8.8 |
This analysis underscores the pressing need for Meta to enhance the Maverick AI model’s capabilities. by focusing on improving key performance indicators, Meta could potentially reposition Maverick AI to compete more effectively in an increasingly crowded AI marketplace.
Strategies for Enhancing Maverick AI’s competitive Edge
To elevate Maverick AI’s standing in the competitive landscape of AI chat models, a multifaceted approach focused on refinement and innovation is essential. emphasizing user experience can directly impact the model’s effectiveness, by integrating feedback loops that enable more tailored interactions. Establishing robust channels for user feedback will allow Meta to identify frequent pain points and areas needing enhancement, ensuring that Maverick’s responses are not only accurate but also resonate on a personal level. Additionally, investing in cutting-edge training techniques, such as reinforcement learning from human feedback, could help Maverick adapt in real-time, further bridging the gap with its competitors.
Moreover, building a strong ecosystem around Maverick AI can foster collaboration and creativity, setting it apart from other models.Key strategies could include:
- Partnerships with Educational Institutions: Collaborating on research to explore advanced linguistics and cognitive science methodologies.
- Open Development framework: Encouraging developers to contribute to Maverick’s codebase, driving community innovation.
- Features focused on Specific Niches: Tailoring services for industries such as healthcare or finance where specialized knowledge can create significant value.
Strategy | Expected outcome |
---|---|
User Feedback Integration | Improved response relevance and user satisfaction |
Reinforcement Learning | Enhanced adaptability and performance over time |
Partnerships with Institutions | access to groundbreaking research and innovative ideas |
Open Development Framework | Community-driven improvements and unique features |
future Development Pathways for Elevating Meta’s AI Models
To enhance Meta’s AI models, especially in the wake of the vanilla Maverick’s performance on competitive benchmarks, a multi-faceted approach is essential. Some proposed pathways include:
- Data Diversification: Expanding the data sets utilized for training to include a more diverse array of conversational contexts can definitely help improve model performance.
- Algorithm Optimization: Focusing on refining the underlying algorithms to improve grasp on contextual nuances and conversational flow can elevate engagement levels.
- Feedback Integration: systematically incorporating user feedback to iteratively adjust and upgrade model responses would facilitate responsiveness to real-world applications.
- cross-Model Learning: Exploring synergies with other AI initiatives within Meta to share learnings and methodologies could provide insights for enhanced development.
Strategically, a collaboration framework with academic institutions and AI research centers may prove beneficial. Consider the following potential endeavors to align research efforts effectively:
Collaboration Type | Potential Benefits |
---|---|
Research Partnerships | access to cutting-edge methodologies and innovative solutions. |
Industry Collaborations | Opportunities for real-world testing and validation of AI applications. |
Open Source Contributions | fostering a community-driven approach to accelerate improvements. |
Final Thoughts
In the ever-evolving landscape of artificial intelligence, where every advancement promises to reshape our understanding of human-computer interaction, Meta’s recent foray with its vanilla Maverick AI model offers a fascinating case study. While the model has garnered attention for its innovative undertakings, its ranking below competitors on a widely recognized chat benchmark raises critically important questions about performance, user experience, and the future of conversational AI. As technology companies race to develop the next generation of bright systems, Maverick’s performance serves as a reminder of the challenges that lie ahead.As we reflect on this latest chapter in AI development, it’s clear that progress is not merely defined by headlines or hype. Instead, it involves continuous refinement, learning from rivals, and responding to user needs in an increasingly competitive marketplace. While the Maverick may not have soared to the top of the rankings this time, its journey is just beginning. The insights gained from this experience could pave the way for future models, as Meta and others strive not just for popularity, but for meaningful engagement in the world of AI-driven dialogue. In this dynamic arena, every setback is an prospect for growth, and the conversation is far from over.