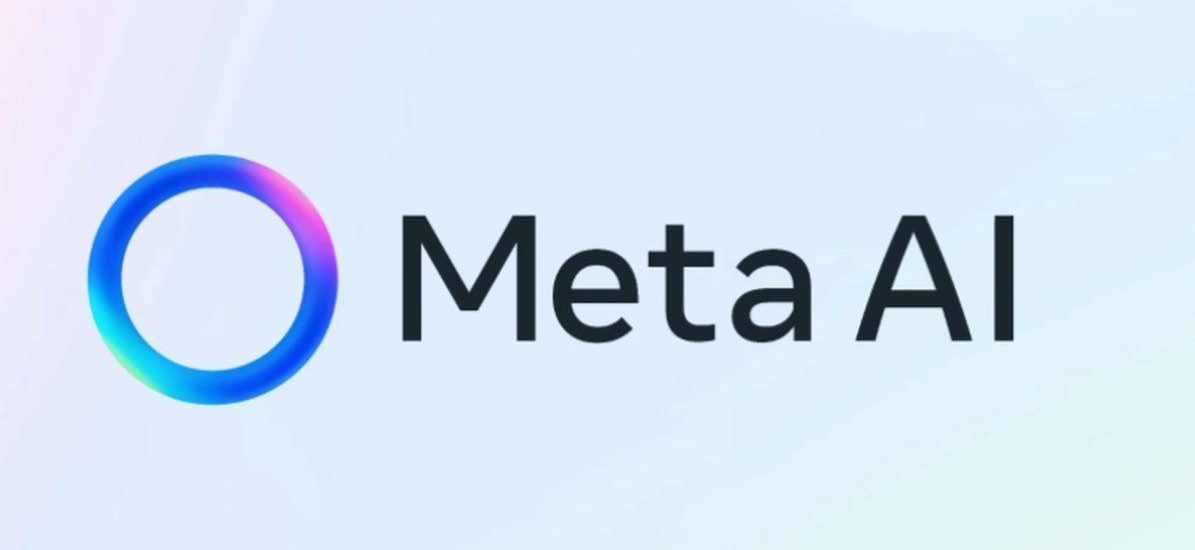
In an era where technology drives our daily interactions adn shapes our understanding of reality, the emergence of artificial intelligence is both a marvel and a concern. Amidst the whirlwind of innovation, Meta, the tech giant formerly known as Facebook, has recently unveiled its AI-powered social feed — a feature promised to enhance user experience by curating content more intelligently. Though, beneath the glossy surface of personalization lies a murky underbelly of privacy risks that warrants closer scrutiny. As we navigate this brave new world of algorithmic engagement,we must ask: is convenience worth the potential compromise of our personal data? In this article,we delve into the implications of Meta’s AI social feed,exploring why it may be a privacy disaster waiting to unfold.
The Implications of Personalized AI Content on user Privacy
The rise of personalized AI content is a beacon of convenience and relevance, yet it casts a long shadow over user privacy. With algorithms meticulously tailored to curate feeds based on individual preferences, the data harvested goes far beyond mere clicks and likes. Users often unwittingly expose sensitive information about their habits, preferences, and even emotional states. Moreover, the vast troves of personal data collected by platforms like Meta can lead to profound implications, including:
- Data Breaches: Centralized repositories of personal data are prime targets for cyberattacks.
- Surveillance Concerns: Constant profiling can lead to invasive monitoring practices.
- Manipulative Advertising: Hyper-targeted ads can exploit vulnerabilities in users’ behavior.
In the relentless pursuit of engaged users, companies may prioritize algorithmic efficiency over robust privacy safeguards, frequently enough resulting in an unsettling trade-off between personalization and personal privacy. This dilemma becomes even more pronounced when considering the opaque nature of data usage policies, which can leave users unsure about how their data is being utilized. To illustrate this tension, consider the following table that contrasts user expectations of privacy with the reality of data collection practices:
User Expectations | Data Collection Reality |
---|---|
Clear data usage policies | Complex terms and conditions |
Control over personal information | Limited opt-out options |
Safe and secure data handling | Frequent data breaches |
Navigating Data Collection: What Users need to Know
As Meta continues to roll out its AI-driven social feed, users are increasingly concerned about how their data will be used and shared. The integration of advanced algorithms means that personal information is not just collected but actively analyzed to curate user experiences. This raises notable privacy risks, as many users remain unaware of what data is being harvested.Key aspects to consider include:
- Data Collection Methods: Understand how data is obtained, from posts to interactions.
- User Consent: Are you aware of what you consented to when signing up?
- Data Storage: Where is yoru data stored, and who has access to it?
In addition to understanding data collection, users should be aware of their rights concerning data protection. Transparency is crucial; platforms should inform users not only about what data is collected but also how it is used. A simplified comparison of potential risks versus the features offered can clarify the importance of vigilance:
Potential Risks | Features Offered |
---|---|
Invasive data tracking | Personalized content recommendations |
Data breaches compromising privacy | Enhanced social connectivity |
Manipulative advertising tactics | Curated advertising experiences |
Building a Safer AI Social feed Through Transparency
In an era where data privacy concerns are at the forefront, the need for transparency in AI-driven social feeds cannot be overstated. Users should be able to see how their data is collected, processed, and used to curate content. Clear guidelines on data usage and an easily accessible privacy policy are essential in building trust between the platform and its users. This transparency can empower individuals to make informed decisions about their online presence and the information they share. The following measures can enhance the transparency of AI social feeds:
- User-Controlled Privacy Settings: Allow users to adjust what data is shared and how it influences their feed.
- Regular Transparency Reports: Provide periodic updates regarding data usage and algorithm adjustments.
- Feedback Mechanisms: Introduce options for users to report inaccuracies in the feed influenced by AI.
Moreover, establishing an ethical framework for AI deployment is crucial to preventing misuse of user data. The implementation of accountability protocols ensures that AI systems are not only user-pleasant but also respect privacy rights at every level. as a step toward this, platforms can integrate an easily understandable transparency dashboard featuring insights into how algorithms personalize content. Consider the following essential components of such a dashboard:
Component | Description |
---|---|
Data Sources | Where user data is sourced from (e.g., interactions, preferences). |
Algorithm Influence | Details on how user data affects feed recommendations. |
User Controls | Options available for users to manage their data. |
Performance Metrics | Effectiveness of content personalization and its impact on user engagement. |
Innovative Approaches to Enhance User Privacy in AI Platforms
As AI platforms like Meta’s social feed gain traction, it’s essential to rethink how user privacy is safeguarded. Implementing decentralized data control could empower users by giving them ownership of their personal information. Some innovative methods include:
- Zero-knowledge proofs: Allowing users to verify their identities without revealing personal data.
- Privacy-preserving machine learning: Enabling models to learn from data without directly accessing it, thus minimizing exposure.
- Anonymized data aggregation: Collecting and processing data in a manner where individual identities remain obscured.
Moreover, adopting a transparent algorithmic approach can enhance trust. By making algorithms comprehensible and understandable to users, platforms can demystify how data is used for content curation. Consider a transparent framework that includes:
Feature | Description |
---|---|
Algorithm Explainability | Providing users with insights into how their data influences feed algorithms. |
User Control Settings | Offering customizable privacy settings that adjust data sharing preferences easily. |
Regular Audits | Conducting periodic reviews to ensure compliance with privacy standards. |
Wrapping Up
while Meta’s AI-driven social feed promises to revolutionize how we engage with content and connect with others, it is crucial to keep a vigilant eye on the potential privacy pitfalls that lie in wait. As algorithms learn more about our behaviors and preferences, the line between personalization and intrusion blurs, raising pressing questions about data ownership and consent. As users, we must advocate for transparency and accountability, ensuring that innovation does not come at the expense of our privacy. The future of our digital interactions may hinge upon how we navigate this delicate balance—striving for a social landscape where technology enhances our lives without compromising our basic rights. As this narrative unfolds, staying informed and proactive will be our best defense against a privacy disaster lurking in the shadows.